Research Showcase
The Paul G. Allen School’s 2025 Annual Research Showcase + Open House will be held in the Bill & Melinda Gates Center for Computer Science & Engineering on Wednesday, October 29, 2025.
Check back soon for 2025 information.
This in-person day allows our affiliates, alumni and friends to meet our students and faculty, via technical talks during the day followed by an open house featuring a reception, poster session, and presentation of the Madrona Prize and People’s Choice Prize in the early evening.
Getting here: Directions, parking and hotel information
During the day – Research Presentations:
The day begins at 10:00 a.m. with registration (and coffee!) in the Singh Gallery, located on the fourth floor of the Gates Center.
- 10:00 a.m. to 10:30 a.m.: Registration (and coffee!)
- 10:30 a.m. to 12:30 p.m.: Technical sessions (see below) in multiple tracks focused on specific themes
- 12:30 to 1:30 p.m.: Keynote talk + Lunch + Networking
- 1:30 to 4:45 p.m.: Technical sessions in multiple tracks focused on specific themes
During the early evening – Open House:
Join us for a festive evening in the Allen Center.
- 5:00-7:15 p.m.: Open house: reception, poster session, and socializing
- 7:15-7:30 p.m.: Presentation of the Madrona Prize and People’s Choice Prize
Research Showcase Agenda
2024 Speaker & Session Details
Schedule of Events – Tuesday, October 29, 2024
Morning
Time: 9:30 – 10:15am
Event: Research Group Affiliates – Coffee and Networking
Location: Various labs
Time: 10:00 – 10:30am
Event: Registration and coffee
Location: Singh Gallery, 4th floor Gates Center
Time: 10:00 – 10:25am
Event: ABET Feedback Session
Location: Zillow Commons, 4th floor Gates Center
Time: 10:30 – 11:10am
Event: Welcome and Overview by Magda Balazinska and Shwetak Patel + various faculty on research areas
Location: Zillow Commons, 4th floor Gates Center
Session I
Time: 11:15am – 12:20pm
Edge and Mobile AI (Gates Center, Room 271)
11:15-11:20: Introduction and Overview, Shyam Gollakota
11:20-11:35: IRIS: Wireless Ring for Vision-based Smart Home Interaction, Maruchi Kim
Integrating cameras into wireless smart rings has been challenging due to size and power constraints. We introduce IRIS, the first wireless vision-enabled smart ring system for smart home interactions. Equipped with a camera, Bluetooth radio, inertial measurement unit (IMU), and an onboard battery, IRIS meets the small size, weight, and power (SWaP) requirements for ring devices. IRIS is context-aware, adapting its gesture set to the detected device, and can last for 16-24 hours on a single charge. IRIS leverages the scene semantics to achieve instance-level device recognition. In a study involving 23 participants, IRIS consistently outpaced voice commands, with a higher proportion of participants expressing a preference for IRIS over voice commands regarding toggling a device’s state, granular control, and social acceptability. Our work pushes the boundary of what is possible with ring form-factor devices, addressing system challenges and opening up novel interaction capabilities.
11:35-11:50: Look Once to Hear: Target Speech Hearing with Noisy Examples, Malek Itani
In crowded settings, the human brain can focus on speech from a target speaker, given prior knowledge of how they sound. We introduce a novel intelligent hearable system that achieves this capability, enabling target speech hearing to ignore all interfering speech and noise, but the target speaker. A naïve approach is to require a clean speech example to enroll the target speaker. This is however not well aligned with the hearable application domain since obtaining a clean example is challenging in real world scenarios, creating a unique user interface problem. We present the first enrollment interface where the wearer looks at the target speaker for a few seconds to capture a single, short, highly noisy, binaural example of the target speaker. This noisy example is used for enrollment and subsequent speech extraction in the presence of interfering speakers and noise. Our system achieves a signal quality improvement of 7.01 dB using less than 5 seconds of noisy enrollment audio and can process 8 ms of audio chunks in 6.24 ms on an embedded CPU. Our user studies demonstrate generalization to real-world static and mobile speakers in previously unseen indoor and outdoor multipath environments. Finally, our enrollment interface for noisy examples does not cause performance degradation compared to clean examples, while being convenient and user-friendly. Taking a step back, this paper takes an important step towards enhancing the human auditory perception with artificial intelligence.
11:50-12:05: Hearable Devices with Sound Bubbles, Tuochao Chen
The human auditory system has a limited ability to perceive distance and distinguish speakers in crowded settings. A headset technology that can create a sound bubble in which all speakers within the bubble are audible, but speakers and noise outside the bubble are suppressed, could augment human hearing. However, developing such technology is challenging. Here we report an intelligent headset system capable of creating sound bubbles. The system is based on real-time neural networks that use acoustic data from up to six microphones integrated into noise-cancelling headsets and are run on-device, processing 8 ms audio chunks in 6.36 ms on an embedded central processing unit. Our neural networks can generate sound bubbles with programmable radii between 1 and 2 meters, and with output signals that reduce the intensity of sounds outside the bubble by 49 decibels. With previously unseen environments and wearers, our system can focus on up to two speakers within the bubble with one to two interfering speakers and noise outside the bubble.
12:05-12:20: Knowledge Boosting: Model Collaboration During Low-Latency Inference, Vidya Srinivas
Models for low-latency, streaming applications could benefit from the knowledge capacity of larger models, but edge devices cannot run these models due to resource constraints. A possible solution is to transfer hints during inference from a large model running remotely to a small model running on-device. However, this incurs a communication delay that breaks real-time requirements and does not guarantee that both models will operate on the same data at the same time. We propose knowledge boosting, a novel technique that allows a large model to operate on time-delayed input during inference, while still boosting small model performance. Using a streaming neural network that processes 8 ms chunks, we evaluate different speech separation and enhancement tasks with communication delays of up to six chunks or 48 ms. Our results show larger gains where the performance gap between the small and large models is wide, demonstrating a promising method for large-small model collaboration for low-latency applications.
Data Management (Gates Center, Room 371)
11:15-11:20: Introduction and Overview, Magda Balazinska
11:20-11:35: Galley: Modern Query Optimization for Sparse Tensor Programs, Kyle Deeds
Tensor programming has become a foundational paradigm for computing in the modern era. It promises an efficient high-level interface for array processing workloads. However, existing frameworks are fundamentally imperative and struggle to optimize workloads involving sparse tensors. This leaves the user in the role of performance engineer, having to make complex algorithmic decisions to achieve reasonable performance. In this talk, I’ll describe Galley, a system for declarative sparse tensor programming which automatically optimizes the user’s program across a variety of levels. At the logical level, Galley optimizes the placement of aggregates and when to materialize intermediates, breaking the program down into a series of sparse tensor kernels (i.e., loop nests). At the physical level, Galley optimizes the loop order of each kernel and the output formats, among other things. Lastly, we’ll show an experimental evaluation which demonstrates the strong performance impact of our optimizations.
11:35-11:50: VegaPlus: Optimizing Dataflow Systems for Scalable Interactive Visualization, Junran Yang
Supporting the interactive exploration of large datasets is a popular and challenging use case for data management systems. Traditionally, the interface and the back-end system are built and optimized separately, and interface design and system optimization require different skill sets that are difficult for one person to master. To enable analysts to focus on visualization design, we contribute VegaPlus, a system that automatically optimizes interactive dashboards to support large datasets. To achieve this, VegaPlus leverages two core ideas. First, we introduce an optimizer that can reason about execution plans in Vega, a back-end DBMS, or a mix of both environments. The optimizer also considers how user interactions may alter execution plan performance and can partially or fully rewrite the plans when needed. Through a series of benchmark experiments on seven different dashboard designs, our results show that VegaPlus provides superior performance and versatility compared to standard dashboard optimization techniques.
11:50-12:05: Query Sketches: Unlocking Knowledge Graph Querying for the Next Million Users, Moe Kayali
Knowledge graphs (KGs) have seen unprecedented uptake by governments and large enterprises but remain difficult to query. The large number of entities (hundreds of millions) and predicates (tens of thousands) makes writing analytical queries on the fly impractical even for expert users. We introduce query sketches, a novel approach for expressing the analyst’s intent in a semi-formal language. This obviates the need for the analyst to know the full schema, while still supporting the full complexity of the underlying query language. We then propose query sketch repair to repair these query sketches into valid queries. This requires learning non-isomorphic mappings. Further, we show that—due to their generality—query sketches also have applications for integrating heterogeneous KGs together.
12:05-12:20: CETUS: A Cost Effective LLM-based Table Understanding System, Guorui Xiao and Lindsey Wei
Web tables are abundant online and are frequently utilized by researchers for downstream tasks such as machine learning model training. However, effectively leveraging these tables requires a semantic understanding of their content—a task known as Table Understanding. While Pretrained Language Models have achieved state-of-the-art performance in this domain, they often require large amounts of well-labeled, task-specific data for training, limiting their generalizability and practicality. Large Language Models (LLMs) offer improvements in these aspects, but serializing entire tables into LLMs is computationally costly and inefficient. In this paper, we propose Celtus, a unified and cost-effective framework for LLM-based Table Understanding that achieves high performance at reduced cost by selectively processing sub-content of tables.
Fundamental AI (Gates Center, Zillow Commons)
11:15-11:20: Introduction and Overview, Noah Smith
11:20-11:40: Tuning Black Box Language Models by Proxy, Alisa Liu
Despite the general capabilities of large pre-trained language models, they consistently benefit from further adaptation to the intended use case. However, tuning these models has become increasingly resource-intensive or completely impossible when model weights are private. We present proxy-tuning, a lightweight decoding-time algorithm that operates on top of black-box LMs to achieve the same end as direct tuning but by accessing only its predictions over the output vocabulary, not its parameters. We show that proxy-tuning closes much of the gap with direct tuning when applied to alignment, domain adaptation, and task-specific fine-tuning. Proxy-tuning has been useful in a number of applications, such as unlearning, multilingual adaptation, and tuning LLMs on on-device data, and it has served as the basis for further work on black-box tuning.
11:40-12:00: Infini-gram: An Efficient Search Engine over Massive Pretraining Corpora of Language Models, Jiacheng (Gary) LiuWeijia Shi
Modern language models (LMs) are pre-trained on massive text corpora consisting of trillions of tokens, and yet we lack a fast and cheap way to understand what these corpora contain. We present infini-gram, an efficient tool to execute counting, searching, and document retrieval queries over massive text corpora. Infini-gram builds a suffix-array index of the corpus, and its engine can answer various types of queries with millisecond-level latency. We apply infini-gram to multiple open pretraining corpora that amount to 5 trillion tokens in total, and consequently, we have built the largest ever n-gram LM and with unbounded n. We show that this unbounded n-gram LM can greatly improve the perplexity of neural, Transformer-based LMs when combined. Infini-gram can be useful in many AI research directions, such as detecting test set contamination, mitigating copyright infringement, curating datasets, evaluating text creativity, and attributing LM outputs to their training data. As such, we have released a web interface and an API endpoint of the tool to the public, and as of October 2024, our service has served over 100M queries.
12:00-12:20: Beyond Monolithic Language Models, Weijia Shi
The current practice of building language models focuses on training huge monolithic models on large-scale data. While scaling the model has been the primary focus of model development, this approach alone does not address several critical issues. For example, LMs often suffer from hallucinations, are difficult to update with new knowledge, and pose copyright and privacy risks. In this talk, I will explore factors important for building models beyond scale. I will discuss augmented LMs—models that access external data or tools during inference to improve reliability. Next, I will explore modular models that maintain data provenance to support takedown requests and unlearning processes.
Lunch + Keynote Talk
Time: 12:25 – 1:25pm
Generative AI for Multimodal Biomedicine, by Sheng Wang
Generative AI for Multimodal Biomedicine, by Sheng Wang, Paul G. Allen School of Computer Science & Engineering (Microsoft Atrium in the Allen Center)
Abstract
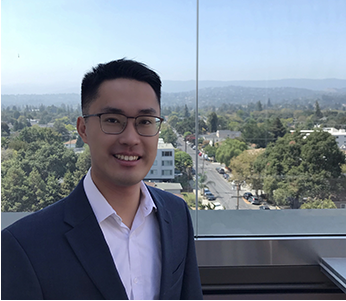
Biomedicine is inherently multimodal, including imaging modalities such as pathology, CT, MRI, X-ray and ultrasounds, as well as omics modality such as genomics, epigenomics and transcriptomics. General domain multimodal approaches are not applicable to biomedicine because biomedical images are very different from general domain images, thus necessitating the development of modality-specific approaches. In this talk, I will introduce three recent works towards building multimodal biomedicine foundation models. First, I will introduce GigaPath, the first whole-slide pathology foundation model that can handle gigapixel-level pathology images. GigaPath exploits a novel vision transformer architecture and achieves the state-of-the-art results on 23 out of 26 cancer tasks, including subtyping and biomarker prediction. Next, I will introduce OCTCube, the first 3D OCT retinal imaging foundation model. OCTCube significantly outperformed 2D models on 27 out of 29 tasks, including retinal disease prediction, cross-modality analysis, cross-device generalization and systemic disease prediction. Finally, I will introduce BiomedParse, a multi-modal foundation model that integrates 9 major biomedical imaging modalities by projecting all of them into the text space, resulting in superior performance on segmentation, detection, and recognition, paving the path for large-scale image-based biomedical discovery. I will conclude this task with discussion on how multi-modal generative AI can advance future medical applications through multi-agent framework and integration with multi-omics datasets.
Bio
Sheng Wang is an assistant professor in the School of Computer Science & Engineering at the University of Washington Seattle. He obtained his B.S. degree in Computer Science from Peking University, Ph.D. degree in Computer Science from University of Illinois at Urbana Champaign, and conducted postdoc training at Stanford School of Medicine. Sheng is currently interested in developing large-scale models for biomedical applications, with a focus on digital pathology, medical imaging foundation models, chromatin structure prediction, and genomics-based drug discovery. His research has been published in top venues such as Nature, Science, Nature Biotechnology, Nature Methods, Nature Machine Intelligence and The Lancet Oncology, and used by major biomedical institutes, including Mayo Clinic, Chan Zuckerberg Biohub, UW Medicine, and Providence genomics.
Session II
Time: 1:30 – 2:35pm
Human-Computer Interaction (Gates Center, Room 271)
1:30-1:35: Introduction and Overview, Katharina Reinecke
1:35-1:50: Individual Differences in AI-Assisted Decision-Making, Katelyn Mei
The integration of Artificial Intelligence (AI) into decision-making processes is reshaping the way individuals and organizations make choices, enhancing speed and precision through AI assistance. However, designing AI systems that people trust and use effectively requires more than just technical enhancement, it involves understanding the human side of the interaction. In this talk, I will present my research findings on how our cognitive patterns—individual decision-making styles—impact users’ interactions with AI suggestions. Based on these findings, I will discuss implications for the future of AI systems that adapt to diverse preferences of individuals.
1:50-2:05: Kindling a Connection Between HCI and Wildfire Management: Understanding Complexities and Challenges of Geospatial Data Use, Nino Migineishvili
Wildfire and forest management increasingly rely on geospatial data to inform measures for the prevention and control of wildfires. Nevertheless, the challenges that arise from untrained domain experts adopting this complex, non-intuitive technology are not well understood. We interviewed $12$ participants in wildfire and forest management to explore both the technical and the sociotechnical nature of these challenges. Our findings reveal that knowledge and data are fragmented across a large number of stakeholders, from governmental decision-makers to local community members. However, (1) lack of efficient communication and knowledge exchange among these diverse stakeholders hinders the acquisition and processing of geospatial data. Moreover, (2) the impact of decisions informed by geospatial technologies are far-reaching, leading to concerns around modeling bias, interpretability, and a desire to engage local communities and the public. These findings inform opportunities for future HCI research to address the needs of stakeholders in this critical domain.
2:05-2:20: How Computing Researchers Perceive Synthetic Multi-Perspective Dialogues to Anticipate Societal Impacts, Rock Pang
There have been increasing calls for computing researchers to consider the negative societal impacts of their work. However, anticipating these impacts remains challenging, as computing researchers often lack diverse perspectives and ethics training. Here, we explore how researchers engage in conversations with diverse conversational agents using a probe – a prototype that supports computing researchers in anticipating the societal impacts of their projects through multi-agent dialogue. Using large language models, our prototype allows users to interact with diverse viewpoints before synthesizing potential negative outcomes of their research projects. Through think-aloud sessions and interviews with 12 participants, we evaluate how they perceive and engage with the prototype’s multiple LLMs when brainstorming about societal impacts, and whether they find it beneficial over using no LLM or only using one LLM. Our findings revealed that participants valued the conversations with stakeholders and considered impacts from new angles. It provides researchers with the insights and agency to reflect on issues rather than passively consuming pre-generated content. We discuss the findings, opportunities, and implications of using multiple LLMs to anticipate societal impact.
2:20-2:35: Dataset Needs and Principles for Human Centered UI Sketch Systems, Sam Ross
We envision a future of software design and development that is multimodal. Instead of only writing code, software designers will be able to sketch what they want their user interfaces (UIs) to look like, and use natural language to specify desired program behavior. Sketch-based UI design tools are a longstanding HCI research topic, with recent work investigating the potential of ML-based approaches. However, current sketch recognition datasets are produced using rigid guidelines for drawing different UI elements. This fails to capture variation in how different people intuitively draw UIs, making tools built using these systems less usable and, potentially, less inclusive. Future systems should be created using data from people drawing UI elements in ways that are intuitive to them. To begin to understand variation in how people intuitively sketch UIs, and develop implications for future sketch-based design tools, we conducted a study of 21 individuals sketching UIs without constraints on their UI element depictions. We find that participants have vast and varied intuitive representations of UI elements and sketching behaviors. We provide five recommendations for how sketch-based UI systems can be more usable through better support of intuitive representational practices.
Social reinforcement learning to align and cooperate with humans (Gates Center, Room 371)
1:30-1:35: Introduction and Overview, Natasha Jaques
1:35-1:55: Personalizing Reinforcement Learning from Human Feedback with Variational Preference Learning, Natasha Jaques
Reinforcement Learning from Human Feedback (RLHF) is a powerful paradigm for aligning foundation models to human values and preferences. However, current RLHF techniques cannot account for the naturally occurring differences in individual human preferences across a diverse population. When these differences arise, traditional RLHF frameworks simply average over them, leading to inaccurate rewards and poor performance for individual subgroups. To address the need for pluralistic alignment, we develop a class of multimodal RLHF methods. Our proposed techniques are based on a latent variable formulation – inferring a novel user-specific latent and learning reward models and policies conditioned on this latent without additional user-specific data. While conceptually simple, we show that in practice, this reward modeling requires careful algorithmic considerations around model architecture and reward scaling. To empirically validate our proposed technique, we first show that it can provide a way to combat underspecification in simulated control problems, inferring and optimizing user-specific reward functions. Next, we conduct experiments on pluralistic language datasets representing diverse user preferences and demonstrate improved reward function accuracy. We additionally show the benefits of this probabilistic framework in terms of measuring uncertainty, and actively learning user preferences. This work enables learning from diverse populations of users with divergent preferences, an important challenge that naturally occurs in problems from robot learning to foundation model alignment.
1:55-2:15: Learning to Cooperate with Humans using Generative Agents, Yancheng Liang
Abstract forthcoming.
2:15-2:35: Infer Human’s Intentions Before Following Natural Language Instructions, Yanming Wan
For AI agents to be helpful to humans, they should be able to follow natural language instructions to complete everyday cooperative tasks in human environments. However, real human instructions inherently possess ambiguity, because the human speakers assume sufficient prior knowledge about their hidden goals and intentions. Standard language grounding and planning methods fail to address such ambiguities because they do not model human internal goals as additional partially observable factors in the environment. We propose a new framework, Follow Instructions with Social and Embodied Reasoning (FISER), aiming for better natural language instruction following in collaborative embodied tasks. Our framework makes explicit inferences about human goals and intentions as intermediate reasoning steps. We implement a set of Transformer-based models and evaluate them over a challenging benchmark, HandMeThat. We empirically demonstrate that using social reasoning to explicitly infer human intentions before making action plans surpasses purely end-to-end approaches. We also compare our implementation with strong baselines, including Chain of Thought prompting on the largest available pre-trained language models, and find that FISER provides better performance on the embodied social reasoning tasks under investigation, reaching the state-of-the-art on HandMeThat.
AI in the Physical World: Robotics (Gates Center, Zillow Commons)
1:30-1:35: Introduction and Overview, Joshua R. Smith
1:35-1:50: The Amazon Science Hub, Joshua R. Smith
I will provide an overview of the Amazon Science Hub, a multi-disciplinary research center at UW founded in 2021. After an overview of the Hub, I will discuss work in the Hub’s largest project, “Manipulation in densely packed containers,” sponsored by Amazon Robotics. The other talks in the session are related to current and future work in the Science Hub.
1:50-2:05: AHA: A Vision-Language Model for Detecting and Reasoning over Failures in Robotic Manipulation, Jiafei Duan
Robotic manipulation in open-world settings demands not only the execution of tasks but also the ability to detect and learn from failures during execution. While recent advances in vision-language models (VLMs) and large language models (LLMs) have enhanced robots’ spatial reasoning and problem-solving capabilities, these models often struggle to recognize and reason about failures, limiting their effectiveness in real-world applications. We introduce AHA, an open-source VLM specifically designed to detect and reason about failures in robotic manipulation through natural language. By framing failure detection as a free-form reasoning task, AHA identifies failures and generates detailed explanations adaptable across various robots, tasks, and environments in both simulation and real-world scenarios. To fine-tune AHA, we developed FailGen, a scalable simulation framework that procedurally generates AHA dataset—the first large-scale dataset of robotic failure trajectories—by perturbing successful demonstrations from the RLBench simulator. Despite being trained solely on the AHA dataset, AHA generalizes effectively to real-world failure datasets, different robotic systems, and unseen tasks. It surpasses the second-best model by 10.3% and exceeds the average performance of all six compared models—including five state-of-the-art VLMs and one model employing in-context learning—by 35.3% across multiple metrics and datasets. Moreover, we integrate AHA into three VLM/LLM-assisted manipulation frameworks. Its natural language failure feedback enhances error recovery and policy performance through methods such as improving reward functions with Eureka reflection, optimizing task and motion planning, and verifying sub-task success in zero-shot robotic manipulation. Our approach achieves an average task success rate 21.4% higher than GPT-4 models.
2:05-2:20: Learning to Grasp in Clutter with Interactive Visual Failure Prediction, Michael Murray
Modern warehouses process millions of unique objects which are often stored in densely packed containers. To automate tasks in this environment, a robot must be able to pick diverse objects from highly cluttered scenes. Real-world learning is a promising approach, but executing picks in the real world is time-consuming, can induce costly failures, and often requires extensive human intervention, which causes operational burden and limits the scope of data collection and deployments. In this work, we leverage interactive probes to visually evaluate grasps in clutter without fully executing picks, a capability we refer to as Interactive Visual Failure Prediction (IVFP). This enables autonomous verification of grasps during execution to avoid costly downstream failures as well as autonomous reward assignment, providing supervision to continuously shape and improve grasping behavior as the robot gathers experience in the real world, without constantly requiring human intervention. Through experiments on a Stretch RE1 robot, we study the effect that IVFP has on performance – both in terms of effective data throughput and success rate, and show that this approach leads to grasping policies that outperform policies trained with human supervision alone, while requiring significantly less human intervention.
2:20-2:35: Data Efficient Behavior Cloning for Fine Manipulation via Continuity-based Corrective Labels, Quinn Pfeifer
We consider imitation learning with access only to expert demonstrations, whose real-world application is often limited by covariate shift due to compounding errors during execution. We investigate the effectiveness of the Continuity-based Corrective Labels for Imitation Learning (CCIL) framework in mitigating this issue for real-world fine manipulation tasks. CCIL generates corrective labels by learning a locally continuous dynamics model from demonstrations to guide the agent back toward expert states. Through extensive experiments on peg insertion and fine grasping, we provide the first empirical validation that CCIL can significantly improve imitation learning performance despite discontinuities present in contact-rich manipulation. We find that: (1) real-world manipulation exhibits sufficient local smoothness to apply CCIL, (2) generated corrective labels are most beneficial in low-data regimes, and (3) label filtering based on estimated dynamics model error enables performance gains. To effectively apply CCIL to robotic domains, we offer a practical instantiation of the framework and insights into design choices and hyperparameter selection. Our work demonstrates CCIL’s practicality for alleviating compounding errors in imitation learning on physical robots.
Session III
Time: 2:40 – 3:45pm
Health & Biology (Gates Center, Room 271)
2:40-2:45: Introduction and Overview, Su-In Lee
2:45-3:00: Medical pedagogy videos as a source of rich multimodal data for semantic, dense, and reasoning tasks, Wisdom O. Ikezogwo
Medical pedagogy videos offer a valuable yet underexplored source of multimodal data, integrating visual, spatial, auditory, and textual elements that encapsulate diverse clinical knowledge. We investigate the potential of medical instructional videos as a source for a robust dataset for a wide range of tasks, including semantic diagnostic understanding, dense spatial tasks, and complex reasoning. By aligning transcripts, image frames, and other metadata, these videos provide a unique opportunity to train models in both specialized and generalized medical knowledge. We propose methods for data preprocessing and curation, multimodal alignment, and representation, with emphasis on the ability of these models to perform medical domain tasks. Our findings suggest that the difficulty of collecting holistic medical multimodal datasets can be easily solved without breaking norms or introducing new constraints to clinician behavior.
3:00-3:15: Towards transparent medical image AI usage via explainable AI, Chanwoo Kim
Building trustworthy and transparent image-based medical artificial intelligence (AI) systems requires the ability to interrogate data and models at all stages of the development pipeline, from training models to post-deployment monitoring. Ideally, the data and associated AI systems could be described using terms already familiar to physicians, but this requires medical datasets densely annotated with semantically meaningful concepts. In the present study, we present a foundation model approach, named MONET (medical concept retriever), which learns how to connect medical images with text and densely scores images on concept presence to enable important tasks in medical AI development and deployment such as data auditing, model auditing and model interpretation. Dermatology provides a demanding use case for the versatility of MONET, due to the heterogeneity in diseases, skin tones and imaging modalities. We trained MONET based on 105,550 dermatological images paired with natural language descriptions from a large collection of medical literature. MONET can accurately annotate concepts across dermatology images as verified by board-certified dermatologists, competitively with supervised models built on previously concept-annotated dermatology datasets of clinical images. We demonstrate how MONET enables AI transparency across the entire AI system development pipeline, from building inherently interpretable models to dataset and model auditing, including a case study dissecting the results of an AI clinical trial.
3:15-3:30: Multi-pass, single-molecule nanopore reading of long protein strands, Daphne Kontogiorgos-Heintz
The ability to sequence single protein molecules in their native, full-length form would enable a more comprehensive understanding of proteomic diversity. Current technologies, however, are limited in achieving this. I will discuss a method for the long-range, single-molecule reading of intact protein strands on the MinION, a commercial nanopore sequencing device intended for nucleic acid sequencing. This method achieves sensitivity to single amino acids on synthetic protein strands hundreds of amino acids in length, enabling the sequencing of combinations of single-amino-acid substitutions and the mapping of post-translational modifications, such as phosphorylation. We also demonstrate the ability to reread individual protein molecules multiple times; to predict raw nanopore signals a priori; and to examine full-length, native folded protein domains. These results provide proof of concept for a platform that has the potential to identify and characterize full-length proteoforms at single-molecule resolution.
3:30-3:45: Generative AI for Retinal Imaging, Zucks Liu
Optical coherence tomography (OCT) has become critical for diagnosing retinal diseases as it enables 3D images of the retina and optic nerve. OCT acquisition is fast, non-invasive, affordable, and scalable. Due to its broad applicability, massive numbers of OCT images have been accumulated in routine exams, making it possible to train large-scale foundation models that can generalize to various diagnostic tasks using OCT images. Nevertheless, existing foundation models for OCT only consider 2D image slices, overlooking the rich 3D structure. Here, we present OCTCube, a 3D foundation model pre-trained on 26,605 3D OCT volumes encompassing 1.62 million 2D OCT images. OCTCube is developed based on 3D masked autoencoders and exploits FlashAttention to reduce the larger GPU memory usage caused by modeling 3D volumes. OCTCube outperforms 2D models when predicting 8 retinal diseases in both inductive and cross-dataset settings, indicating that utilizing the 3D structure in the model instead of 2D data results in significant improvement. OCTCube further shows superior performance on cross-device prediction and when predicting systemic diseases, such as diabetes and hypertension, further demonstrating its strong generalizability. Finally, we propose a contrastive-self-supervised-learning-based OCT-IR pre-training framework (COIP) for cross-modality analysis on OCT and infrared retinal (IR) images, where the OCT volumes are embedded using OCTCube. We demonstrate that COIP enables accurate alignment between OCT and IR en face images. Collectively, OCTCube, a 3D OCT foundation model, demonstrates significantly better performance against 2D models on 27 out of 29 tasks and comparable performance on the other two tasks, paving the way for AI-based retinal disease diagnosis.
HCI and AI in Health and Accessibility (Gates Center, Room 371)
2:40-2:45: Introduction and Overview, James Fogarty
2:45-3:00: MigraineTracker: Examining Patient Experiences with Goal-Directed Self-Tracking for a Chronic Health Condition, Yasaman S. Sefidgar
Self-tracking and personal informatics offer important potential in chronic condition management, but such potential is often undermined by difficulty in aligning self-tracking tools to an individual’s goals. Informed by prior proposals of goal-directed tracking, we designed and developed MigraineTracker, a prototype app that emphasizes explicit expression of goals for migraine-related self-tracking. We then examined migraine patient experiences in a deployment study for an average of 12+ months, including a total of 50 interview sessions with 10 patients working with 3 different clinicians. Patients were able to express multiple types of goals, evolve their goals over time, align tracking to their goals, personalize their tracking, reflect in the context of their goals, and gain insights that enabled understanding, communication, and action. We discuss how these results highlight the importance of accounting for distinct and concurrent goals in personal informatics together with implications for the design of future goal-directed personal informatics tools.
3:00-3:15: From Classification to Clinical Insights: Towards Analyzing and Reasoning About Mobile and Behavioral Health Data With Large Language Models, Zachary Englhardt
Passively collected behavioral health data from ubiquitous sensors could provide mental health professionals valuable insights into patient’s daily lives, but such efforts are impeded by disparate metrics, lack of interoperability, and unclear correlations between the measured signals and an individual’s mental health. To address these challenges, we pioneer the exploration of large language models (LLMs) to synthesize clinically relevant insights from multi-sensor data. We develop chain-of-thought prompting methods to generate LLM reasoning on how data pertaining to activity, sleep and social interaction relate to conditions such as depression and anxiety. We then prompt the LLM to perform binary classification, achieving accuracies of 61.1%, exceeding the state of the art. We find models like GPT-4 correctly reference numerical data 75% of the time. While we began our investigation by developing methods to use LLMs to output binary classifications for conditions like depression, we find instead that their greatest potential value to clinicians lies not in diagnostic classification, but rather in rigorous analysis of diverse self-tracking data to generate natural language summaries that synthesize multiple data streams and identify potential concerns. Clinicians envisioned using these insights in a variety of ways, principally for fostering collaborative investigation with patients to strengthen the therapeutic alliance and guide treatment. We describe this collaborative engagement, additional envisioned uses, and associated concerns that must be addressed before adoption in real-world contexts.
3:15-3:30: ExerciseRx: Supporting Exercise Prescription as a Medicine using Generative AI, Richard Li
Physical activity can be a powerful tool for combating chronic health conditions and for supporting general wellness. However, patient adherence to exercise goals is low, and provider burden in prescribing exercises is high. In this work, we present the ExerciseRx ecosystem for supporting both patients and providers in this process. First, we show how a patient-facing mobile app providing real-time feedback on their exercises increases adherence to fitness goals. Then, we demonstrate a tool for supporting providers in prescribing personalized exercise plans. We discuss how closing the loop on this patient/provider ecosystem will lead to improving medical outcomes.
3:30-3:45: The Ability-Based Design Mobile Toolkit (ABD-MT): Developer Support for Runtime Interface Adaptation Based on Users’ Abilities, Judy Kong
Despite significant progress in the capabilities of mobile devices and applications, most apps remain oblivious to their users’ abilities. To enable apps to respond to users’ situated abilities, we created the Ability-Based Design Mobile Toolkit (ABD-MT). ABD-MT integrates with an app’s user input and sensors to observe a user’s touches, gestures, physical activities, and attention at runtime, to measure and model these abilities, and to adapt interfaces accordingly. Conceptually, ABD-MT enables developers to engage with a user’s “ability profile,” which is built up over time and inspectable through our API. As validation, we created example apps to demonstrate ABD-MT, enabling ability-aware functionality in 91.5% fewer lines of code compared to not using our toolkit. Further, in a study with 11 Android developers, we showed that ABD-MT is easy to learn and use, is welcomed for future use, and is applicable to a variety of end-user scenarios.
Panel: AI Safety (Gates Center, Zillow Commons)
2:40-2:45: Introduction and Overview Hila Gonen
2:45-3:35: Interactive discussion
Panelists: Hila Gonen; Liwei Jiang; Inna Lin
Session IV
Time: 3:50 – 4:55pm
AI Systems & Infrastructure (Gates Center, Room 271}
3:50-3:55: Introduction and Overview, Arvind Krishnamurthy
3:55-4:10: Palu: KV-Cache Compression with Low-Rank Projection, Chien-Yu Lin
Post-training KV-Cache compression methods often sample effectual tokens or quantize data, but they miss redundancy in the hidden dimension of KV-Cache. In this work, we introduce Palu, a KV-Cache compression framework that utilizes low-rank projection to reduce the hidden dimension of KV tensors, and cut LLM memory usage at inference. To avoid the expensive online decomposition cost, Palu decomposes the weight matrices of the linear layers of Key and Value, cache the compressed latent representation and reconstruct full keys and values on the fly. To achieve strong accuracy and fast decoding time, Palu incorporates (1) medium-grained low-rank decomposition, (2) efficient rank search, (3) low-rank-aware quantization, and (4) optimized GPU kernels. Extensive experiments show that, for RoPE-based LLMs, Palu with 50% low-rank compression and 4-bit quantization delivers up to 2.91x and 2.59x speedup on the attention module and end-to-end runtime, largely exceeding the speed of quantization-only methods while maintaining strong accuracy. For non-RoPE LLMs, Palu further pushes the speedup of attention and end-to-end latency to up to 6.17x and 5.53x.
4:10-4:25: ForestColl: Efficient Collective Communications on Heterogeneous Network Fabrics, Liangyu Zhao
As modern DNN models grow ever larger, collective communications between the accelerators (allreduce, etc.) emerge as a significant performance bottleneck. Designing efficient communication schedules is challenging, given today’s highly diverse and heterogeneous network fabrics. In this paper, we present ForestColl, a tool that generates performant schedules for any network topology. ForestColl constructs broadcast/aggregation spanning trees as the communication schedule, achieving theoretically optimal throughput. Its schedule generation runs in strongly polynomial time and is highly scalable. ForestColl supports any network fabric, including both switching fabrics and direct connections. We evaluated ForestColl on multi-box AMD MI250 and NVIDIA DGX A100 platforms. ForestColl’s schedules delivered up to 130% higher performance compared to the vendors’ own optimized communication libraries, RCCL and NCCL, and achieved a 20% speedup in LLM training. ForestColl also outperforms other state-of-the-art schedule generation techniques with both up to 61% more efficient generated schedules and orders of magnitude faster schedule generation speed.
4:25-4:40: NanoFlow: Towards Optimal Large Language Model Serving Throughput, Kan Zhu
As the demand for serving large language models (LLMs) at a planet-scale continues to surge, optimizing throughput under latency constraints has become crucial for GPU-based serving systems. Existing solutions primarily focus on inter-device parallelism (e.g., data, tensor, pipeline parallelism), but fail to fully exploit the available resources within a single device, leading to under-utilization and performance bottlenecks. In this talk, we introduce NanoFlow, a novel serving framework designed to maximize intra-device parallelism. By splitting inference requests into nano-batches at the granularity of individual operations, NanoFlow enables overlapping of compute, memory, and network resources within a single GPU. This is achieved through operation-level pipelining and execution unit scheduling, allowing different operations to run simultaneously in distinct functional units. NanoFlow’s automated parameter search mechanism further simplifies its adaptation to diverse models. Our implementation on NVIDIA GPUs demonstrates significant performance gains, providing a 1.91× throughput boost compared to state-of-the-art methods across several popular LLMs like LLaMA-2-70B and Qwen2-72B achieving 59% to 72% of the optimal throughput.
4:40-4:55: FlashInfer: High-Performant and Customizable Attention Kernels for LLM Serving, Zihao Ye
Transformers power large language models (LLMs), making efficient GPU attention kernels essential for fast inference. Existing attention kernel libraries either underperform or fail to generalize to new attention variants. We introduce FlashInfer, a unified framework that optimizes attention computation with a block-sparse format for memory efficiency and a customizable JIT-compiled attention template. FlashInfer also features dynamic scheduling to handle runtime variability, while supporting CUDAGraph. FlashInfer is open-sourced at FlashInfer and have been adopted by leading LLM serving engines such as vLLM, sglang and MLC-LLM, FlashInfer delivers significant performance improvements in both kernel-level and end-to-end evaluations.
Graphics and Vision (Gates Center, Room 371 )
3:50-3:55: Introduction and Overview, Ira Kemelmacher-Shlizerman
3:55-4:07: Inverse Painting: Reconstructing The Painting Process, Bowei Chen
Given an input painting, we reconstruct a time-lapse video of how it may have been painted.
4:07-4:19: Title forthcoming, Johanna Karras
Abstract forthcoming.
4:19-4:31: Task Me Anything, Jieyu Zhang
We introduce Task-Me-Anything, a benchmark generation engine which produces a benchmark for evaluating multimodal language models tailored to a user’s needs.
4:31-4:43: Generative In-betweening: Adapting Image-to-Video Diffusion Models for Keyframe Interpolation, Xiaojuan Wang
Given a pair of key frames as input, our method generates a continuous intermediate video with coherent motion by adapting a a pretrained image-to-video diffusion model.
4:43-4:55: Computational Illusion Knitting, Amy Zhu
Illusion-knit fabrics reveal hidden images across viewing angles. Artist-created knit illusions are tedious to design, limited to single-view, and slow to manufacture. We establish constraints over the design space, develop an interactive design system, and originate fabrication techniques for mixed colorwork and texture, successfully creating the first known double-view illusions.
AI in the Physical World: Sustainability in the environment (Gates Center, Zillow Commons)
3:50-3:55: Introduction and Overview, Vikram Iyer
3:55-4:15: ProxiCycle: Passively Mapping Cyclist Safety Using Smart Handlebars for Near-Miss Detection, Joe Breda
If the global population leveraged cycling for 2.6 kilometers (1.6 miles) a day (the current average in the Netherlands), global emissions from passenger vehicles would drop by 20%. This is significant as transportation by personal passenger car is repeatedly found to be the largest single contributor to transportation sector’s greenhouse gas (GHG) emissions with the transportation sector being the largest portion of total GHG emissions worldwide. This motivates mode switching from car-based to cycling (or other active transit like walking or public transport) as one of the most direct approaches an individual can take to better their own health and reduce their environmental impact. The primary factors preventing cyclist adoption are safety concerns, specifically, the fear of collision from automobiles. One solution to address this concern is to direct cyclists to known safe routes to minimize risk and stress, thus making cycling more approachable. However, few localized safety priors are available, hindering safety based routing. Specifically, road user behavior is unknown. To address this issue, we develop a novel handlebar attachment to passively monitor the proximity of passing cars as a an indicator of cycling safety along historically traveled routes. We deploy this sensor with 15 experienced cyclists in a 2 month longitudinal study to source a citywide map of car passing distance. We then compare this signal to both historic collisions and perceived safety reported by experienced and inexperienced cyclists.
4:15-4:35: Incorporating Sustainability in Electronics Design: Obstacles and Opportunities, Felix Hähnlein
Life cycle assessment (LCA) is a methodology to holistically measure the environmental impact of a product from initial manufacturing through end-of-life disposal. However, it is currently unclear to what extent LCA informs the design of computing devices. To understand how this information is collected and utilized, we interviewed 17 industry professionals with LCA or electronics design experience, systematically coded the interviews, and investigated common themes. The themes reveal that gathering data is a key LCA challenge and depict distributed decision-making processes where it is often unclear who is responsible for sustainable design decisions and at what cost. Our analysis reveals opportunities for HCI technologies to support LCA computation and LCA integration into the design process to inform sustainability-oriented decision making. While this work focuses on nuanced discussion about sustainable design in the information and communication technologies (ICT) hardware industry, we hope our insights are also valuable for other sectors.
Evening
Time: 5:00 – 7:00pm
Event: Open House: Reception + Poster Session
Location: Microsoft Atrium in the Allen Center
Time: 7:15 – 7:30pm
Event: Madrona Prize, People’s Choice Awards
Location: Microsoft Atrium in the Allen Center