When imagining the future of technology, sometimes all we need to do is look out the window — or into a microscope.
Our researchers take inspiration from nature to redefine what a computer can be, from data storage using synthetic DNA, to sensors modeled on insects and leaves. We also advance technologies to help solve biology’s biggest mysteries, such as computational approaches for understanding the mechanisms of disease and brain-computer interfaces that can restore or augment physical function and mobility.
Research Groups & Labs
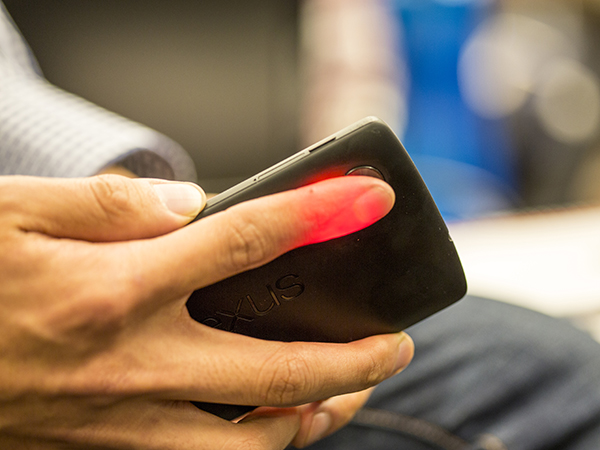
UbiComp Lab
The Ubiquitous Computing (UbiComp) Lab develops innovative systems for health sensing, low-power sensing, energy sensing, activity recognition and novel user interface technology for real-world applications.
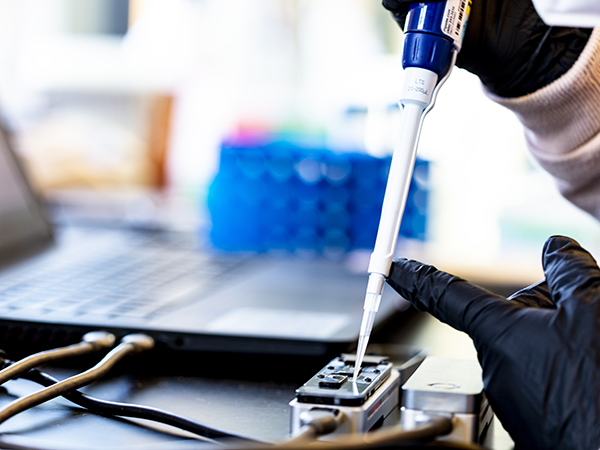
Molecular Information Systems Lab (MISL)
MISL explores the intersection of information technology and molecular biology using in-silico and wet lab experiments, drawing upon expertise from computer architecture, programming languages, synthetic biology and biochemistry.
Faculty Members
Centers & Initiatives

Computing for the Environment (CS4Env)
Computing for the Environment (CS4Env) at the University of Washington supports novel collaborations across the broad fields of environmental sciences and computer science & engineering. The initiative engages environmental scientists and engineers, computer scientists and engineers, and data scientists in using advanced technologies, methodologies and computing resources to accelerate research that addresses pressing societal challenges related to climate change, pollution, biodiversity and more.
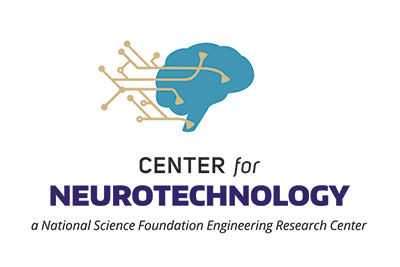
Center for Neurotechnology (CNT)
The Center for Neurotechnology (CNT) got its start in 2011 as one of several Engineering Research Centers (ERCs) funded by the National Science Foundation. CNT is headquartered at the University of Washington, with core partners at the Massachusetts Institute of Technology and San Diego State University. CNT researchers focus on developing and applying principles of engineered neuroplasticity to revolutionize the treatment of spinal cord injury, stroke and other debilitating neurological conditions.
Highlights
Agents of Tech
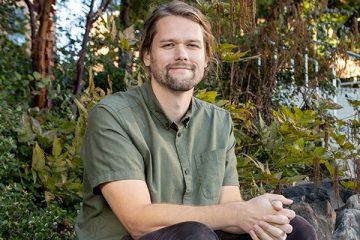
Nivala, co-director of the Molecular Information Systems Lab (MISL), discusses the groundbreaking potential of DNA-based data storage and its role in the future of AI.
Allen School News
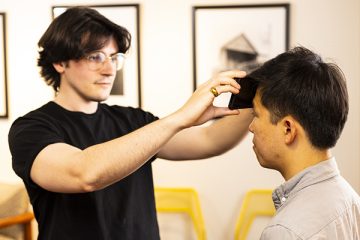
Researchers in the UbiComp Lab and UW Medicine earned an IMWUT Distinguished Paper Award for their work on an app that turns a smartphone into a thermometer.
UW News
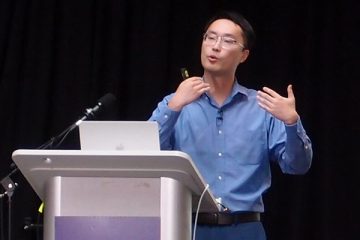
In this Q&A, Allen School professor Sheng Wang talks about his work on a new medical AI model, BiomedParse, that works across nine different types of medical images to better predict systemic diseases. Clinicians can load images into the system and ask questions in plain English.